Static Image
Generated rotational face
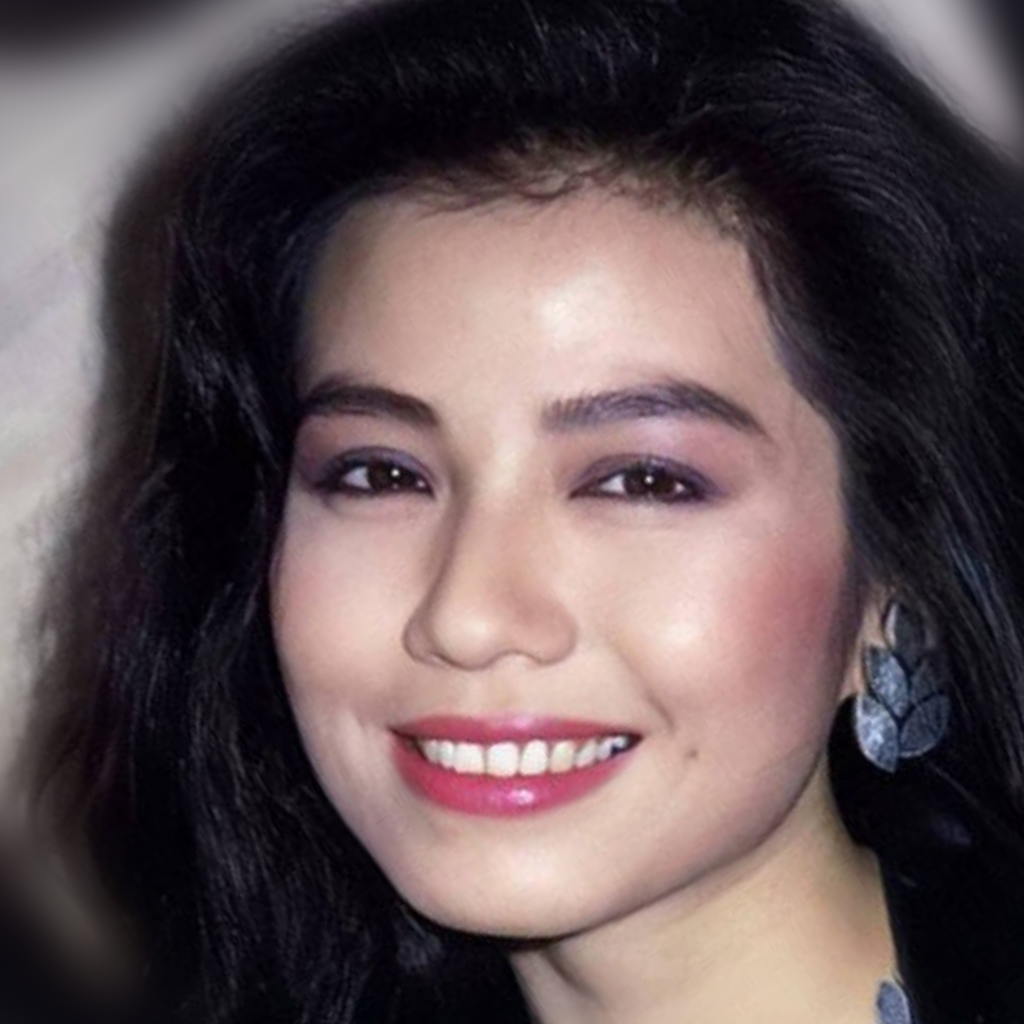
1st Demo: This is a video demonstration to illustrate the effect of the above paper.
This static image was trained to rotate at different angle on the right. The eye-blinking effect is introduced to enhance interest of readers. It was not created by the model. (The static image on the left-hand side is a picture taken in the wild.)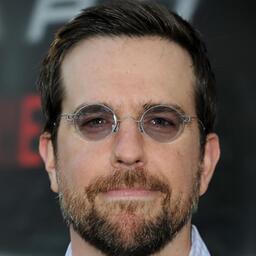
2nd Demo
(The static image on the left-hand side is sourced from the CelebA-HQ dataset.)Abstract
Manipulating facial poses is challenging, especially when addressing significant pose variations. While extensive research has been dedicated to address large poses and manipulate various facial expressions, this frequently results in compromised image quality. The challenge may arise from nonlinearity of the latent space. We must navigate a complex path along the high-quality image manifold and determine the optimal direction for the face rotation task, which may secure the most effective disentanglement. Moreover, the regularity of the latent space also affects directly the quality of the resulting image. In this paper, we have made a careful study of the latent space, and deliberately crafted our model to identify the complicated trajectory of rotating facial manipulation with exceptional disentanglement. Our facial pose generative model, aims at enhancing the quality of generated images while preserving the identity and fidelity and achieving better disentanglement. Data acquisition is another challenging aspect, requiring extensive preparation and meticulous setup. To address this, we suggest a flipping technique to mitigate dataset limitations. Ultimately, we strive to strike a balance between image quality and pose generation, ensuring that our results are both visually pleasing and accurately representing the desired facial pose.
The Architecture
Our primary methodology focuses on manipulating the latent space of StyleGAN, leveraging its well-structured and versatile nature. By incorporating additional pathways within the latent space, we extend its functionality to include facial pose adjustments. The resultant images benefit from the high-quality synthesis capabilities of the StyleGAN model, ensuring realistic and detailed outcomes.
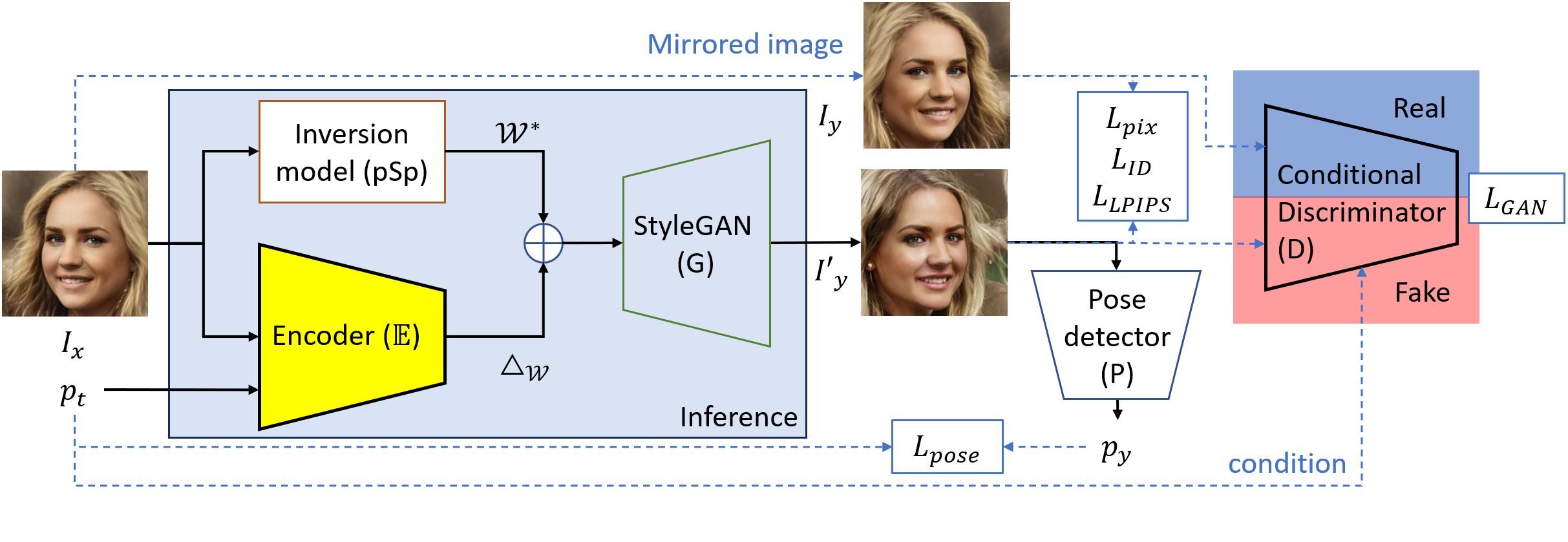
Our main contributions are summarized as follows:
- We propose an unsupervised learning approach for pose manipulation through latent space manipulation. This approach eliminates the need to prepare ground truth images in training by utilizing a face-flipping strategy.
- The model explicitly separates the learning of identity representation from the features of facial pose, enabling it to produce high-quality image of desired pose.
- Our architecture utilizes an encoder and the StyleGAN generator to synthesize the image. We also use a conditional discriminator to restrict the generated images conforming it within the facial domain. In order to ensure that the generated face features align with the original image, the back projection technique or the Back projection loss can be used. This architecture improves the quality of the generated image while preserving its identity.
- The proposed model demonstrates outstanding image quality when generating facial poses on CelebA-HQ and LFW datasets. The high-quality images generated can effectively be utilized in various forms of creative entertainment and enhance the recognition rate of facial recognition systems. In the experimental results, our methods have verified that our approach give remarkable results compared with other approaches.