Static Image
Description
Personalized Talking Style
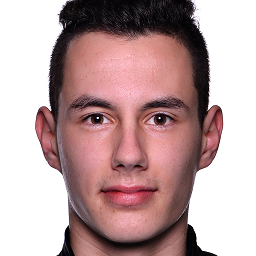
This static image was trained to talk like the reference video on the right.
Play the video on the right to see the simulated personalized talking video of the static image.
To compare the generated with the original, click
here
(Note that the video has been super-resoluted)
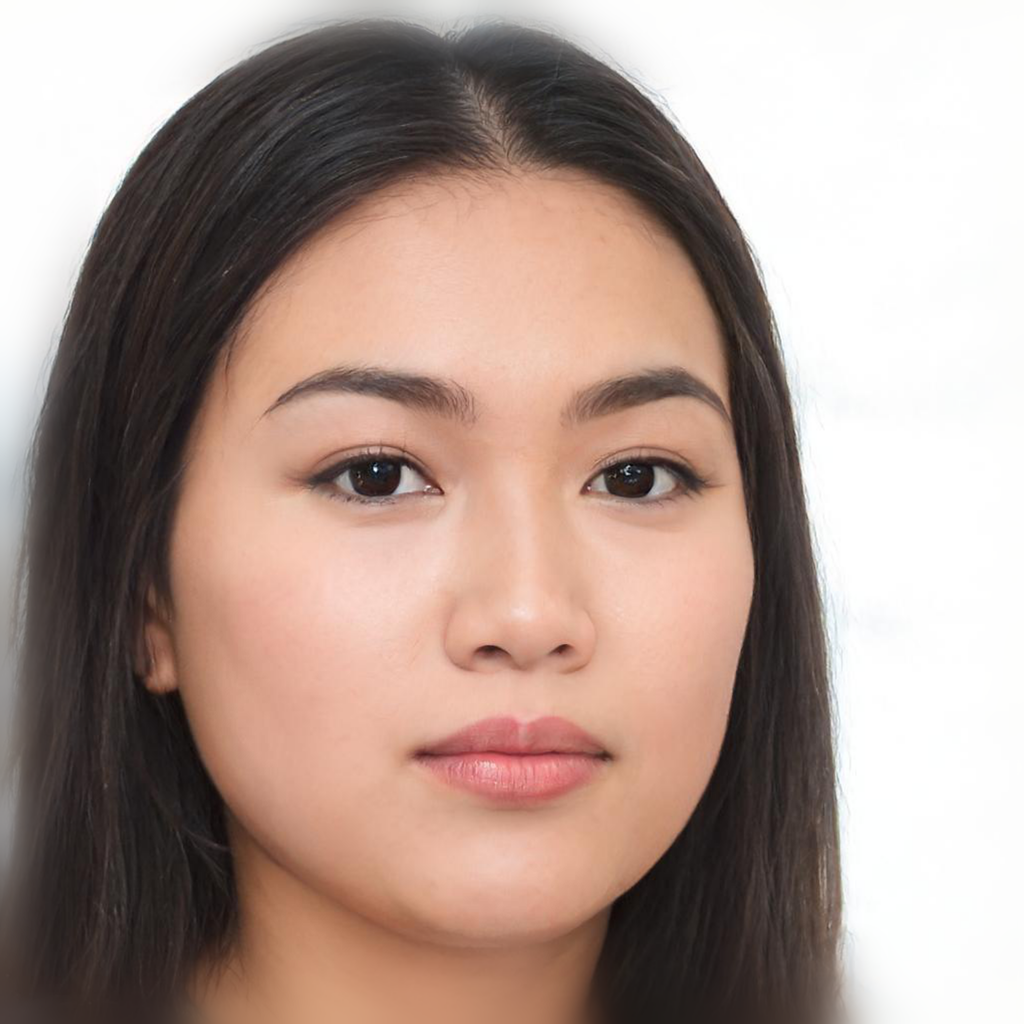
This static image was trained to talk like the reference video on the right.
Play the video on the right to see the simulated personalized talking video of the static image.
To compare the generated with the original, click
here
(Note that the video has been super-resoluted)
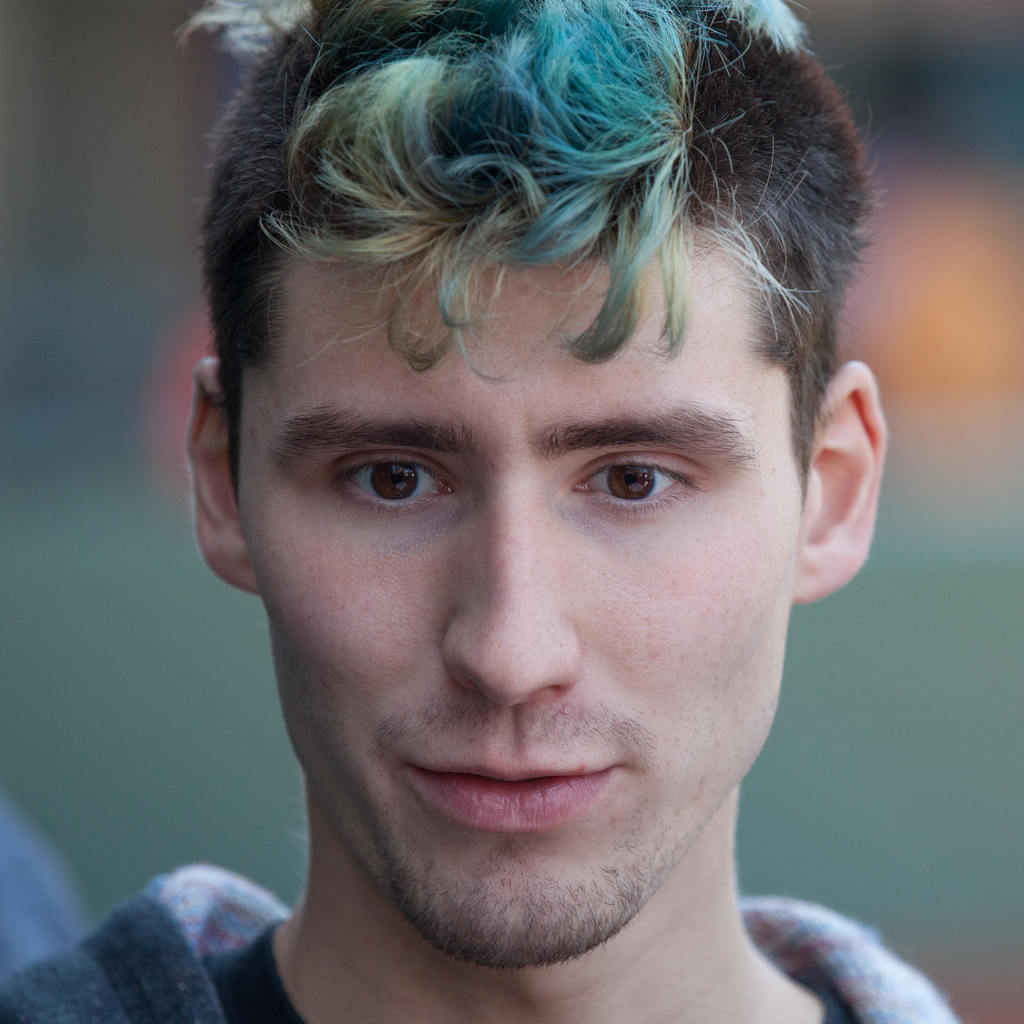
(Note that the video has been super-resoluted)
Friends, Romans, countrymen, lend me your ears;
I come to bury Caesar, not to praise him.
The evil that men do lives after them;
The good is oft interred with their bones;
So let it be with Caesar. The noble Brutus
Hath told you Caesar was ambitious:
If it were so, it was a grievous fault,
And grievously hath Caesar answer’d it.
Here, under leave of Brutus and the rest–
For Brutus is an honourable man;
So are they all, all honourable men–
Come I to speak in Caesar’s funeral.
He was my friend, faithful and just to me:
But Brutus says he was ambitious;
And Brutus is an honourable man.
He hath brought many captives home to Rome
Whose ransoms did the general coffers fill:
Did this in Caesar seem ambitious?
When that the poor have cried, Caesar hath wept:
Ambition should be made of sterner stuff:
Abstract
This paper focuses on generating photo-realistic talking face videos by leveraging on semantic facial attributes in a latent space and capturing the talking style from an old video of a speaker. We formulate a process to manipulate facial attributes in the latent space by identifying semantic facial directions. We develop a deep learning pipeline to learn the correlation between the audio and the corresponding video frames from a reference video of a speaker in an aligned latent space. This correlation is used to navigate a static face image into frames of a talking face video, which is moderated by three carefully constructed loss functions, for accurate lip synchronization and photo-realistic video reconstruction. By combining these techniques, we aim to generate high-quality talking face videos that are visually realistic and synchronized with the provided audio input. Our results were evaluated against some state-of-the-art techniques on talking face generation, and we have recorded significant improvements in the image quality of the generated talking face video.
The Architecture
Our proposed model consists of three (3) encoders: audio encoder Eaudio, image encoder Eimage and style encoder Estyle On the left-hand side, the first row is the Eaudio encoder for input audio. The second row is the Eimage encoder for inputting static image whereas the last row is Estyle encoder for reference video input. On the right-hand side, comparison is done between the ground truth and generated video frames.
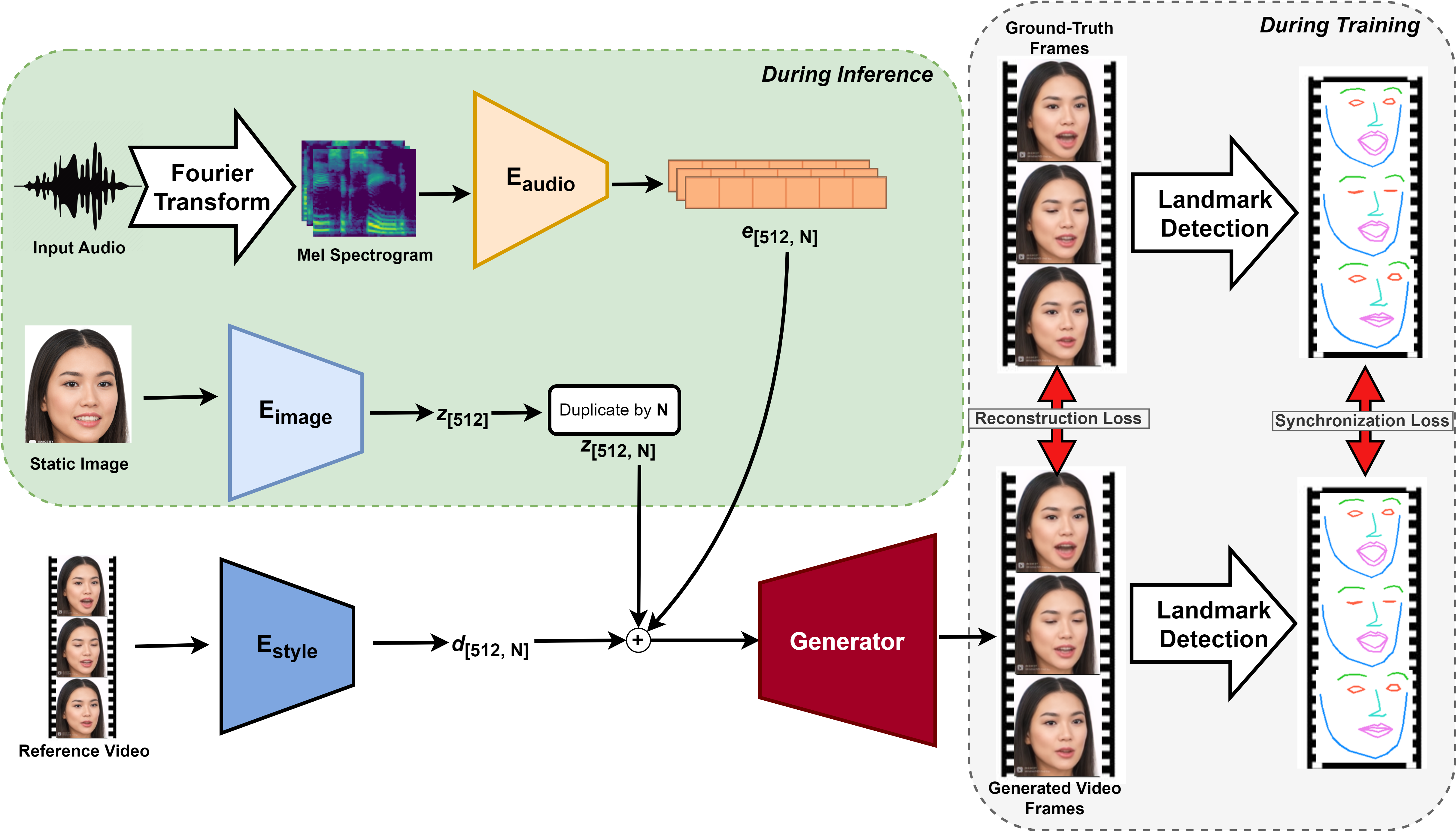
Our specific contributions are summarized as follows:
- We formulate an algorithm to get semantic facial attributes in a latent space. These attributes could be mouth direction in the latent, such that it can be added to any arbitrary latent code (representing a particular face) to give a new latent code that will generate the same face with the mouth open or closed based on the magnitude of the manipulation.
- We utilize the old video of a speaker to capture their talking style by learning the correlation between their speech segments and visual facial styles in an aligned latent space.
- We propose an end-to-end deep learning pipeline to detect motion directions from consecutive frames of a reference video in the form of motion latent variables, and use the variables to navigate the latent code of a static face image into frames of a talking face video.
- We device three loss functions (synchronization, reconstruction and contrastive losses) as the learning schemes that handles accurate lip synchronization using the audio input and the video reference for photo-realistic video reconstruction.